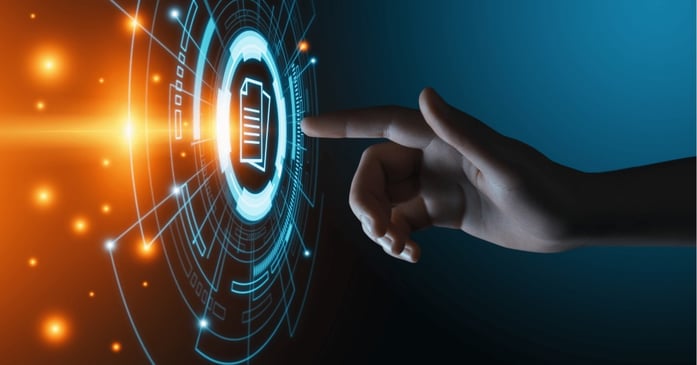
Understanding Predictive Information Governance
- By:
- Bill Tolson |
- March 4, 2020 |
- minute read
Machine Learning and Information Governance
Question: Can your information governance system automatically determine the difference between the term “Penguin” in reference to the flightless bird, the publishing house, or a comic book villain? We are reaching the point where machine learning-based information management systems can do this. If machine learning is capable of contextual comprehension, what could it mean for your organization’s records management and information governance capabilities?
Information Governance and the Human Nature Factor
One of the biggest hassles for records managers continues to be getting employees to recognize data that should be saved in the first place. That doesn’t even begin to factor in the need to correctly categorize it, and file the individual documents, emails, files, and records for compliance, litigation, and business purposes in the correct applications and repositories. Having worked as a consultant for many years, helping companies fix their records management and eDiscovery processes, I have seen the issues associated with relying on individual employees to consistently follow complex information management policies. This is the human nature factor. Getting thousands of individual employees to follow complex information management policies, in the same way, is impossible based on hundreds or thousands of variables. This issue gets even more cumbersome and complex every year with the continuing growth of corporate information that employees must deal with.
The fact is that most of a company’s employees were not trained nor hired to be records managers. It’s additional work they probably don’t get credit for and in many companies, the processes are complex and time consuming.
There is also a challenge in records management called the “five-second rule.” Employees will, on average, spend no more than five seconds referencing, classifying and storing documents as a record. If the process takes longer, even well-meaning employees will ignore retention processes and either tag them as “delete immediately” or “save forever.” Bottom line: the traditional process of looking up a record in a complex retention schedule, dragging the document to a repository, and selecting a classification often exceeds the five-second rule, meaning the records retention schedule is mostly useless.
A few years ago, I was interviewing a group of employees for a consulting engagement. They made the point that if they spent the required time to review, look up the suggested retention period, and move the files/email to the designated repository (figuring 100 to 300 new emails and files per day), they would not have time to actually get even 10% of their “real” work done. This is the reason records managers have been waiting for truly accurate machine learning-based auto-categorization to save them!
Read our Blog: Most Employees are Terrible at Information Management | ![]()
|
Many Variables Affect Manual Document Categorization
Yesterday’s “cross your fingers” approach to information management is illustrated by the eDiscovery review process in the legal industry.
Law firms have long relied on outsourcing document review to contract lawyers to determine if millions of documents are potentially relevant to a given lawsuit. Law firms would hire 5,10,20 or more contract attorneys to manually review millions of documents for relevance to the case. In the legal industry, this manual review process is called linear review. In linear review situations, eDiscovery teams manually analyze documents for key terms and relevance until all potentially relevant documents have been reviewed and tagged for production.
One study found that accuracy rates varied dramatically based on attorney variables such as the country they were born in, the law school they graduated from, if they were married, the ages of their kids, what they had for dinner the night before, etc. Relevancy accuracy rates ranged from 40% to 60% - not something to write home about. The contract attorneys are equivalent to the thousands of employees trying to categorize individual documents for records management properly. It's all dependent on human nature.
Machine Learning-based Predictive Coding in the Legal Industry
In the early 2000s, a new technology emerged in the legal industry called Predictive Coding or Technology Assisted Review (TAR). This machine learning technology was able to take millions of documents and decide which ones were actually relevant to a given case – with accuracy rates in the 90% + range. And, it could do so in hours versus the manual human review, which could take weeks or months.
As you can imagine, many attorneys were not enamored with this new technology. Law firms and attorneys made lots of money on the eDiscovery review process, and if it could be dramatically sped up, then billable hours would drop. Also, most attorneys and judges are usually not considered early technology adopters, so were afraid of this new technology. The acceptance of predictive coding was slow to take off because there was a great deal of anticipation among legal practitioners on how the courts would respond.
Federal Magistrate Judge Andrew Peck's decision in Da Silva Moore v. Publicis Groupe 287 F.R.D. 182 (S.D.N.Y. 2012), is considered the first official judicial endorsement of predictive coding as a way to review documents and started the acceleration of its use. Judge Peck’s ruling concludes that “computer-assisted review is an available tool and should be seriously considered for use in large-data-volume cases where it may save the producing party (or both parties) significant amounts of legal fees in document review.” Today, most judges agree that predictive coding is a great time, and money-saving tool and now has a well-established place in eDiscovery.
Predictive Coding for Information Governance
Machine learning-based or predictive information governance, as opposed to “dumb” keyword-based or department-based information governance, is a data management solution to search out and automatically categorize (and file) data based on content and context within the enterprise. Today’s intelligent information management systems migrate data if needed, categorize and organize it using machine learning to understand the information in context, apply retention/disposition policies, apply access and content controls, anonymize/redact it if needed, and store it appropriately.
Predictive information governance facilitates integrated information governance, eDiscovery, and regulatory compliance by providing a technology-driven transition from reactive, manual information management and eDiscovery processing to proactive, automated data governance.
Harnessing machine learning for information governance enables the automatic categorization and management of unstructured data without the need to rely on individual employees, thus increasing retention/disposition accuracy rates and reducing eDiscovery and compliance risk, enabling enterprises to manage huge amounts of information, accurately and cost-effectively. Put another way, a predictive information governance capability can provide the ability to identify and locate content from a variety of data sources that are similar in meaning and associate them with a specific governance policy for retention, migration, or disposal purposes.
Some organizations have begun to explore the benefits of leveraging these technologies for information governance purposes. For example, what if a machine learning-based cloud information management and archiving system could continuously search out aging files on all department file shares and automatically determine if a given file contains personally identifiable information? And, if so, move it to the cloud archive, apply updated access controls, apply new retention/disposition policies based on its content, and delete the original on the file share? Or, what if the system could automatically journal all emails from the company Office 365 tenant and accurately categorize each email with its appropriate retention period in the cloud archive? The outcome of machine learning-based information governance is extremely accurate information handling, retention, and security while relieving employees of the additional work and responsibility. This is the type of new capabilities records managers have been waiting for.
The Cloud, Machine Learning and AI, and the Benefits of Economies of Scale
Until recently, machine learning and AI for information governance have been emerging technologies that most organizations have not been able to take advantage of because of the high cost of getting into it, lack of in-depth knowledge, and a lack of industry focus. However, the growth of hyper-scale cloud platforms and the integration of machine learning/AI into their services platforms that application vendors can take advantage of has changed everything. Customers can now take advantage of the economies of scale to begin dipping their toes into the machine learning-based information governance waters.
With the industry acceptance of the top three hyper-scale, multi-tenancy clouds - Microsoft Azure, Amazon AWS, and the Google Cloud, machine learning and AI for predictive information governance are becoming a cost-effective capability.
Machine learning and the Microsoft Azure Cloud
In January of 2015, Microsoft purchased a leading predictive coding provider named Equivio. The Equivio technology applies machine learning to help solve the problems of data classification at scale, enabling users to explore large, unstructured data sets and quickly find which ones contain relevant information. It uses advanced text analytics to perform multi-dimensional analyses of data collections, to intelligently sort documents into categories, group near-duplicates, isolate unique data, and help users quickly identify the documents they need. As part of this process, users train the system, using examples of relevant and non-relevant content, to automatically identify documents relevant to a particular subject, such as a specific records class, access rights, if specific documents contain personal information or regulated content, for a legal case, or regulatory investigation. This iterative training, referred to as supervised learning, is much more accurate and cost-effective than manual keyword searches and manual review of vast quantities of documents. Microsoft has built this technology into its Office 365 E5 platform for advanced eDiscovery and has also added it to the Azure services stack.
As I mentioned previously, the technology has achieved broad acceptance in the legal community as a valuable eDiscovery tool, but the question is, can it also be accepted into the information management community?
Machine Learning Use Cases
For example, the financial services (FinServ) industry has very prescriptive data retention and handling requirements for broker/dealers. These include a Supervision requirement – FINRA Rule 3110. This rule requires FinServ firms to establish, maintain, and enforce a system to supervise target employee activities and the activities of their recipients that are “reasonably” designed to achieve compliance with federal securities laws and regulations. Generally accepted requirements include the need to collect all communications from the target employees and review them for non-compliant content. If communications are tagged as being out of compliance, then supervisors must review the communications and determine if they are appropriate or not. Additionally, most FinServ companies want to include a sampling function that automatically collects random communications for supervisor review.
These data sets can become very large over the workday, putting huge workloads on the supervisors. But, what if broker/dealer communications could be automatically analyzed by a predictive supervision capability significantly reducing the numbers of communications flagged by a simple keyword search down to a much smaller data set that supervisors must review? This would amount to huge time savings while also more quickly flagging only those communications that are truly non-compliant.
Another example in the information management and archiving sector is the use of machine learning (ML) and analytics that can be used for both day-forward tasks such as archiving as well as assist with a historical categorization of information in email and other unstructured files. Machine learning offers the ability to structure currently unstructured data and apply information management policies - accurately. Predictive filing utilizes the ML capabilities to support the proactive filing of information, or auto-classification, during its lifecycle.
In fact, any indexable repository, such as archives, share drives, and employee workstations can benefit from this technology. Specifically, it can find and protect PI for the many current and emerging privacy regulations, create relationships between documents based on content and context, and tag these documents for later search, i.e. find all documents that contain company intellectual property and bar them from being sent outside the enterprise or, find all documents that should be tagged for a litigation hold.
Predictive information governance means no more relying on employees to review and accurately file the hundreds of emails and documents they receive per day, no more inaccurate retention periods applied to regulated records, no more inaccurate and risky search results because of faulty manual tagging, no more inaccurate filings based on individual employee deviations, and no more “5-second rule,” blind records filing. The fact is, machine learning-based information governance amounts to the holy grail in the information management industry.
Predictive Information Governance and Archive360
Archive360 can provide you with a solution today that enables you to take advantage of the Predictive Information Governance technology. To find out more about machine learning-based information management, contact Archive360 to discuss the latest updates in this exciting technology.
Bill is the Vice President of Global Compliance for Archive360. Bill brings more than 29 years of experience with multinational corporations and technology start-ups, including 19-plus years in the archiving, information governance, and eDiscovery markets. Bill is a frequent speaker at legal and information governance industry events and has authored numerous eBooks, articles and blogs.